R package citation, R package reverse dependencies, R package scholars, install an r package from GitHub hy is package acceptance pending why is package undeliverable amazon why is package on hold dhl tour packages why in r package r and r package full form why is r free why r is bad which r package to install which r package has which r package which r package version which r package readxl which r package ggplot which r package fread which r package license where is package.json where is package-lock.json where is package.swift where is package explorer in eclipse where is package where is package manager unity where is package installer android where is package manager console in visual studio who r package which r package to install which r package version who is package who is package deal who is package design r and r package full form r and r package meaning what r package has what package r what is package in java what is package what is package-lock.json what is package in python what is package.json what is package installer do r package can't install r packages r can't find package r can't load package can't load xlsx package r can't install psych package r can't install sf package r Write if else in NONMEM pk pd
planningML
View on CRAN: Click
here
Download and install planningML package within the R console
Install from CRAN:
install.packages("planningML")
Install from Github:
library("remotes")
install_github("cran/planningML")
Install by package version:
library("remotes")
install_version("planningML", "1.0.1")
Attach the package and use:
library("planningML")
Maintained by
Xinying Fang
[Scholar Profile | Author Map]
[Scholar Profile | Author Map]
All associated links for this package
10.32614/CRAN.package.planningML . planningML results . planningML.pdf . planningML User Guide . planningML_1.0.1.tar.gz . planningML_1.0.1.zip . planningML_1.0.1.zip . planningML_1.0.1.zip . planningML_1.0.1.tgz . planningML_1.0.1.tgz . planningML_1.0.1.tgz . planningML_1.0.1.tgz . planningML archive . https://CRAN.R-project.org/package=planningML .
First Published: 2022-11-08
Latest Update: 2023-06-23
Description:
Advances in automated document classification has led to identifying massive numbers of clinical concepts from handwritten clinical notes. These high dimensional clinical concepts can serve as highly informative predictors in building classification algorithms for identifying patients with different clinical conditions, commonly referred to as patient phenotyping. However, from a planning perspective, it is critical to ensure that enough data is available for the phenotyping algorithm to obtain a desired classification performance. This challenge in sample size planning is further exacerbated by the high dimension of the feature space and the inherent imbalance of the response class. Currently available sample size planning methods can be categorized into: (i) model-based approaches that predict the sample size required for achieving a desired accuracy using a linear machine learning classifier and (ii) learning curve-based approaches (Figueroa et al. (2012) ) that fit an inverse power law curve to pilot data to extrapolate performance. We develop model-based approaches for imbalanced data with correlated features, deriving sample size formulas for performance metrics that are sensitive to class imbalance such as Area Under the receiver operating characteristic Curve (AUC) and Matthews Correlation Coefficient (MCC). This is done using a two-step approach where we first perform feature selection using the innovated High Criticism thresholding method (Hall and Jin (2010) ), then determine the sample size by optimizing the two performance metrics. Further, we develop software in the form of an R package named 'planningML' and an 'R' 'Shiny' app to facilitate the convenient implementation of the developed model-based approaches and learning curve approaches for imbalanced data. We apply our methods to the problem of phenotyping rare outcomes using the MIMIC-III electronic health record database. We show that our developed methods which relate training data size and performance on AUC and MCC, can predict the true or observed performance from linear ML classifiers such as LASSO and SVM at different training data sizes. Therefore, in high-dimensional classification analysis with imbalanced data and correlated features, our approach can efficiently and accurately determine the sample size needed for machine-learning based classification.
How to cite:
Xinying Fang (2022). planningML: A Sample Size Calculator for Machine Learning Applications in Healthcare. R package version 1.0.1, https://cran.r-project.org/web/packages/planningML. Accessed 07 May. 2025.
Previous versions and publish date:
1.0.0 (2022-11-08 11:20)
Other packages that cited planningML R package
View planningML citation profile
Other R packages that planningML depends,
imports, suggests or enhances
Complete documentation for planningML
Functions, R codes and Examples using
the planningML R package
Some associated functions: featureselection . fit_learningcurve . learningcurve_data . plot.planningML . samplesize . summary.planningML .
Some associated R codes: calculate_PCC_by_DS_Updated.R . featureselection.R . fit_learningcurve.R . learningcurve_data.R . plot.planningML.R . samplesize.R . summary.planningML.R . Full planningML package functions and examples
Downloads during the last 30 days
Today's Hot Picks in Authors and Packages
funLBM
The funLBM algorithm allows to simultaneously cluster the rows and the columns of a data matrix wher ...
Download / Learn more Package Citations See dependency
Download / Learn more Package Citations See dependency
Maintainer: Charles Bouveyron (view profile)
humanize
An almost direct port of the 'python' 'humanize' package .
Thi ...
Download / Learn more Package Citations See dependency
Download / Learn more Package Citations See dependency
Maintainer: Gerry Manoim (view profile)
quickcode
The NOT functions, 'R' tricks and a compilation of some simple quick plus often used 'R' codes to im ...
Download / Learn more Package Citations See dependency
Download / Learn more Package Citations See dependency
Maintainer: Obinna Obianom (view profile)
aroma.affymetrix
A cross-platform R framework that facilitates processing of any number of Affymetrix microarray samp ...
Download / Learn more Package Citations See dependency
Download / Learn more Package Citations See dependency
Maintainer: Henrik Bengtsson (view profile)
MLDS
Difference scaling is a method for scaling perceived
supra-threshold differences. The package cont ...
Download / Learn more Package Citations See dependency
Download / Learn more Package Citations See dependency
Maintainer: Kenneth Knoblauch (view profile)
24,205
R Packages
207,311
Dependencies
65,312
Author Associations
24,206
Publication Badges
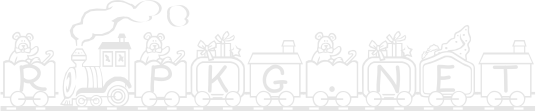