R package citation, R package reverse dependencies, R package scholars, install an r package from GitHub hy is package acceptance pending why is package undeliverable amazon why is package on hold dhl tour packages why in r package r and r package full form why is r free why r is bad which r package to install which r package has which r package which r package version which r package readxl which r package ggplot which r package fread which r package license where is package.json where is package-lock.json where is package.swift where is package explorer in eclipse where is package where is package manager unity where is package installer android where is package manager console in visual studio who r package which r package to install which r package version who is package who is package deal who is package design r and r package full form r and r package meaning what r package has what package r what is package in java what is package what is package-lock.json what is package in python what is package.json what is package installer do r package can't install r packages r can't find package r can't load package can't load xlsx package r can't install psych package r can't install sf package r Write if else in NONMEM pk pd
smoothedLasso
View on CRAN: Click
here
Download and install smoothedLasso package within the R console
Install from CRAN:
install.packages("smoothedLasso")
Install from Github:
library("remotes")
install_github("cran/smoothedLasso")
Install by package version:
library("remotes")
install_version("smoothedLasso", "1.6")
Attach the package and use:
library("smoothedLasso")
Maintained by
Georg Hahn
[Scholar Profile | Author Map]
[Scholar Profile | Author Map]
All associated links for this package
First Published: 2020-04-03
Latest Update: 2021-03-21
Description:
We provide full functionality to smooth L1 penalized regression operators and to compute regression estimates thereof. For this, the objective function of a user-specified regression operator is first smoothed using Nesterov smoothing (see Y. Nesterov (2005) <doi:10.1007/s10107-004-0552-5>), resulting in a modified objective function with explicit gradients everywhere. The smoothed objective function and its gradient are minimized via BFGS, and the obtained minimizer is returned. Using Nesterov smoothing, the smoothed objective function can be made arbitrarily close to the original (unsmoothed) one. In particular, the Nesterov approach has the advantage that it comes with explicit accuracy bounds, both on the L1/L2 difference of the unsmoothed to the smoothed objective functions as well as on their respective minimizers (see G. Hahn, S.M. Lutz, N. Laha, C. Lange (2020) <doi:10.1101/2020.09.17.301788>). A progressive smoothing approach is provided which iteratively smoothes the objective function, resulting in more stable regression estimates. A function to perform cross validation for selection of the regularization parameter is provided.
How to cite:
Georg Hahn (2020). smoothedLasso: A Framework to Smooth L1 Penalized Regression Operators using Nesterov Smoothing. R package version 1.6, https://cran.r-project.org/web/packages/smoothedLasso
Previous versions and publish date:
Other packages that cited smoothedLasso R package
View smoothedLasso citation profile
Other R packages that smoothedLasso depends,
imports, suggests or enhances
Functions, R codes and Examples using
the smoothedLasso R package
Some associated functions: crossvalidation . elasticNet . fusedLasso . graphicalLasso . minimizeFunction . minimizeSmoothedSequence . objFunction . objFunctionGradient . objFunctionSmooth . objFunctionSmoothGradient . prsLasso . standardLasso .
Some associated R codes: Full smoothedLasso package functions and examples
Downloads during the last 30 days
Get rewarded with contribution points by
helping add
Reviews / comments / questions /suggestions ↴↴↴
Today's Hot Picks in Authors and Packages
gclus
Orders panels in scatterplot matrices and parallel coordinate
displays by some merit index. Package ...
Download / Learn more Package Citations See dependency
Download / Learn more Package Citations See dependency
Maintainer: Catherine Hurley (view profile)
nextGenShinyApps
Nove responsive tools for designing and developing 'Shiny' dashboards and applications. The scripts ...
Download / Learn more Package Citations See dependency
Download / Learn more Package Citations See dependency
Maintainer: Obinna Obianom (view profile)
MatrixEQTL
Matrix eQTL is designed for fast eQTL analysis on large datasets.
Matrix eQTL can test for associat ...
Download / Learn more Package Citations See dependency
Download / Learn more Package Citations See dependency
Maintainer: Andrey A Shabalin (view profile)
ismev
Functions to support the computations carried out in
`An Introduction to Statistical Modeling of Ex ...
Download / Learn more Package Citations See dependency
Download / Learn more Package Citations See dependency
Maintainer: Eric Gilleland (view profile)
CompoundEvents
Tools for extracting occurrences, assessing potential driving factors, predicting occurrences, and q ...
Download / Learn more Package Citations See dependency
Download / Learn more Package Citations See dependency
Maintainer: Zengchao Hao (view profile)
multiocc
Spatio-temporal multivariate occupancy models can handle multiple species in occupancy models. This ...
Download / Learn more Package Citations See dependency
Download / Learn more Package Citations See dependency
Maintainer: Staci Hepler (view profile)
22,114
R Packages
188,080
Dependencies
55,244
Author Associations
22,115
Publication Badges
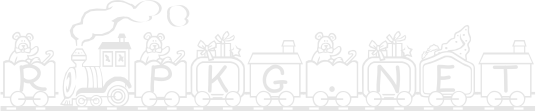
© Copyright 2022 - present. All right reserved, rpkg.net. Contact Us / Suggestions / Concerns