R package citation, R package reverse dependencies, R package scholars, install an r package from GitHub hy is package acceptance pending why is package undeliverable amazon why is package on hold dhl tour packages why in r package r and r package full form why is r free why r is bad which r package to install which r package has which r package which r package version which r package readxl which r package ggplot which r package fread which r package license where is package.json where is package-lock.json where is package.swift where is package explorer in eclipse where is package where is package manager unity where is package installer android where is package manager console in visual studio who r package which r package to install which r package version who is package who is package deal who is package design r and r package full form r and r package meaning what r package has what package r what is package in java what is package what is package-lock.json what is package in python what is package.json what is package installer do r package can't install r packages r can't find package r can't load package can't load xlsx package r can't install psych package r can't install sf package r Write if else in NONMEM pk pd
skedastic
View on CRAN: Click
here
Download and install skedastic package within the R console
Install from CRAN:
install.packages("skedastic")
Install from Github:
library("remotes")
install_github("cran/skedastic")
Install by package version:
library("remotes")
install_version("skedastic", "2.0.2")
Attach the package and use:
library("skedastic")
Maintained by
Thomas Farrar
[Scholar Profile | Author Map]
[Scholar Profile | Author Map]
All associated links for this package
First Published: 2020-01-10
Latest Update: 2024-01-08
Description:
Implements numerous methods for testing for, modelling, and correcting for heteroskedasticity in the classical linear regression model. The most novel contribution of the package is found in the functions that implement the as-yet-unpublished auxiliary linear variance models and auxiliary nonlinear variance models that are designed to estimate error variances in a heteroskedastic linear regression model. These models follow principles of statistical learning described in Hastie (2009) <doi:10.1007/978-0-387-21606-5>. The nonlinear version of the model is estimated using quasi-likelihood methods as described in Seber and Wild (2003, ISBN: 0-471-47135-6). Bootstrap methods for approximate confidence intervals for error variances are implemented as described in Efron and Tibshirani (1993, ISBN: 978-1-4899-4541-9), including also the expansion technique described in Hesterberg (2014) <doi:10.1080/00031305.2015.1089789>. The wild bootstrap employed here follows the description in Davidson and Flachaire (2008) <doi:10.1016/j.jeconom.2008.08.003>. Tuning of hyper-parameters makes use of a golden section search function that is modelled after the MATLAB function of Zarnowiec (2022) <https://www.mathworks.com/matlabcentral/fileexchange/25919-golden-section-method-algorithm>. A methodological description of the algorithm can be found in Fox (2021, ISBN: 978-1-003-00957-3). There are 25 different functions that implement hypothesis tests for heteroskedasticity. These include a test based on Anscombe (1961) <https://projecteuclid.org/euclid.bsmsp/1200512155>, Ramsey's (1969) BAMSET Test <doi:10.1111/j.2517-6161.1969.tb00796.x>, the tests of Bickel (1978) <doi:10.1214/aos/1176344124>, Breusch and Pagan (1979) <doi:10.2307/1911963> with and without the modification proposed by Koenker (1981) <doi:10.1016/0304-4076(81)90062-2>, Carapeto and Holt (2003) <doi:10.1080/0266476022000018475>, Cook and Weisberg (1983) <doi:10.1093/biomet/70.1.1> (including their graphical methods), Diblasi and Bowman (1997) <doi:10.1016/S0167-7152(96)00115-0>, Dufour, Khalaf, Bernard, and Genest (2004) <doi:10.1016/j.jeconom.2003.10.024>, Evans and King (1985) <doi:10.1016/0304-4076(85)90085-5> and Evans and King (1988) <doi:10.1016/0304-4076(88)90006-1>, Glejser (1969) <doi:10.1080/01621459.1969.10500976> as formulated by Mittelhammer, Judge and Miller (2000, ISBN: 0-521-62394-4), Godfrey and Orme (1999) <doi:10.1080/07474939908800438>, Goldfeld and Quandt (1965) <doi:10.1080/01621459.1965.10480811>, Harrison and McCabe (1979) <doi:10.1080/01621459.1979.10482544>, Harvey (1976) <doi:10.2307/1913974>, Honda (1989) <doi:10.1111/j.2517-6161.1989.tb01749.x>, Horn (1981) <doi:10.1080/03610928108828074>, Li and Yao (2019) <doi:10.1016/j.ecosta.2018.01.001> with and without the modification of Bai, Pan, and Yin (2016) <doi:10.1007/s11749-017-0575-x>, Rackauskas and Zuokas (2007) <doi:10.1007/s10986-007-0018-6>, Simonoff and Tsai (1994) <doi:10.2307/2986026> with and without the modification of Ferrari, Cysneiros, and Cribari-Neto (2004) <doi:10.1016/S0378-3758(03)00210-6>, Szroeter (1978) <doi:10.2307/1913831>, Verbyla (1993) <doi:10.1111/j.2517-6161.1993.tb01918.x>, White (1980) <doi:10.2307/1912934>, Wilcox and Keselman (2006) <doi:10.1080/10629360500107923>, Yuce (2008) <https://dergipark.org.tr/en/pub/iuekois/issue/8989/112070>, and Zhou, Song, and Thompson (2015) <doi:10.1002/cjs.11252>. Besides these heteroskedasticity tests, there are supporting functions that compute the BLUS residuals of Theil (1965) <doi:10.1080/01621459.1965.10480851>, the conditional two-sided p-values of Kulinskaya (2008) <doi:10.48550/arXiv.0810.2124>, and probabilities for the nonparametric trend statistic of Lehmann (1975, ISBN: 0-816-24996-1). For handling heteroskedasticity, in addition to the new auxiliary variance model methods, there is a function to implement various existing Heteroskedasticity-Consistent Covariance Matrix Estimators from the literature, such as those of White (1980) <doi:10.2307/1912934>, MacKinnon and White (1985) <doi:10.1016/0304-4076(85)90158-7>, Cribari-Neto (2004) <doi:10.1016/S0167-9473(02)00366-3>, Cribari-Neto et al. (2007) <doi:10.1080/03610920601126589>, Cribari-Neto and da Silva (2011) <doi:10.1007/s10182-010-0141-2>, Aftab and Chang (2016) <doi:10.18187/pjsor.v12i2.983>, and Li et al. (2017) <doi:10.1080/00949655.2016.1198906>.
How to cite:
Thomas Farrar (2020). skedastic: Handling Heteroskedasticity in the Linear Regression Model. R package version 2.0.2, https://cran.r-project.org/web/packages/skedastic. Accessed 07 Nov. 2024.
Previous versions and publish date:
Other packages that cited skedastic R package
View skedastic citation profile
Other R packages that skedastic depends,
imports, suggests or enhances
Complete documentation for skedastic
Functions, R codes and Examples using
the skedastic R package
Some associated functions: GSS . T_alpha . alvm.fit . anlvm.fit . anscombe . avm.ci . avm.fwls . avm.vcov . bamset . bickel . blus . bootlm . breusch_pagan . carapeto_holt . cook_weisberg . countpeaks . dDtrend . diblasi_bowman . dpeak . dpeakdat . dufour_etal . evans_king . glejser . godfrey_orme . goldfeld_quandt . harrison_mccabe . harvey . hccme . hetplot . honda . horn . li_yao . pDtrend . pRQF . ppeak . rackauskas_zuokas . simonoff_tsai . szroeter . twosidedpval . verbyla . white . wilcox_keselman . yuce . zhou_etal .
Some associated R codes: GSS.R . alvm.fit.R . anlvm.fit.R . anscombe.R . avm.ci.R . avm.fwls.R . avm.vcov.R . avmutils.R . bamset.R . bickel.R . blus.R . bootlm.R . breusch_pagan.R . carapeto_holt.R . cook_weisberg.R . data.R . diblasi_bowman.R . dufour_etal.R . evans_king.R . glejser.R . globals.R . godfrey_orme.R . goldfeld_quandt.R . harrison_mccabe.R . harvey.R . hccme.R . hetplot.R . honda.R . horn.R . li_yao.R . myutils.R . pRQF.R . peaks.R . rackauskas_zuokas.R . simonoff_tsai.R . szroeter.R . trend.R . twosidedpval.R . verbyla.R . white.R . wilcox_keselman.R . yuce.R . zhou_etal.R . Full skedastic package functions and examples
Downloads during the last 30 days
Get rewarded with contribution points by
helping add
Reviews / comments / questions /suggestions ↴↴↴
Today's Hot Picks in Authors and Packages
nextGenShinyApps
Nove responsive tools for designing and developing 'Shiny' dashboards and applications. The scripts ...
Download / Learn more Package Citations See dependency
Download / Learn more Package Citations See dependency
Maintainer: Obinna Obianom (view profile)
robregcc
We implement the algorithm estimating the parameters of the robust regression model with composition ...
Download / Learn more Package Citations See dependency
Download / Learn more Package Citations See dependency
Maintainer: Aditya Mishra (view profile)
bacondecomp
Decomposition for differences-in-differences with variation in
treatment timing from Goodman-Bacon ...
Download / Learn more Package Citations See dependency
Download / Learn more Package Citations See dependency
Maintainer: Evan Flack (view profile)
con2aqi
To calculate the AQI (Air Quality Index) from pollutant concentration data.
O3, PM2.5, PM10, CO, SO ...
Download / Learn more Package Citations See dependency
Download / Learn more Package Citations See dependency
Maintainer: Zhicheng Du (view profile)
23,092
R Packages
198,677
Dependencies
62,675
Author Associations
23,089
Publication Badges
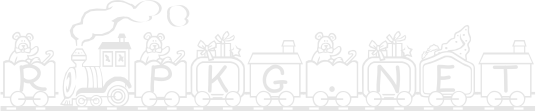