R package citation, R package reverse dependencies, R package scholars, install an r package from GitHub hy is package acceptance pending why is package undeliverable amazon why is package on hold dhl tour packages why in r package r and r package full form why is r free why r is bad which r package to install which r package has which r package which r package version which r package readxl which r package ggplot which r package fread which r package license where is package.json where is package-lock.json where is package.swift where is package explorer in eclipse where is package where is package manager unity where is package installer android where is package manager console in visual studio who r package which r package to install which r package version who is package who is package deal who is package design r and r package full form r and r package meaning what r package has what package r what is package in java what is package what is package-lock.json what is package in python what is package.json what is package installer do r package can't install r packages r can't find package r can't load package can't load xlsx package r can't install psych package r can't install sf package r Write if else in NONMEM pk pd
shattering
View on CRAN: Click
here
Download and install shattering package within the R console
Install from CRAN:
install.packages("shattering")
Install from Github:
library("remotes")
install_github("cran/shattering")
Install by package version:
library("remotes")
install_version("shattering", "1.0.7")
Attach the package and use:
library("shattering")
Maintained by
Rodrigo F. de Mello
[Scholar Profile | Author Map]
[Scholar Profile | Author Map]
All associated links for this package
First Published: 2020-09-25
Latest Update: 2021-08-21
Description:
The Statistical Learning Theory (SLT) provides the theoretical background to ensure that a supervised algorithm generalizes the mapping f:X -> Y given f is selected from its search space bias F. This formal result depends on the Shattering coefficient function N(F,2n) to upper bound the empirical risk minimization principle, from which one can estimate the necessary training sample size to ensure the probabilistic learning convergence and, most importantly, the characterization of the capacity of F, including its under and overfitting abilities while addressing specific target problems. In this context, we propose a new approach to estimate the maximal number of hyperplanes required to shatter a given sample, i.e., to separate every pair of points from one another, based on the recent contributions by Har-Peled and Jones in the dataset partitioning scenario, and use such foundation to analytically compute the Shattering coefficient function for both binary and multi-class problems. As main contributions, one can use our approach to study the complexity of the search space bias F, estimate training sample sizes, and parametrize the number of hyperplanes a learning algorithm needs to address some supervised task, what is specially appealing to deep neural networks. Reference: de Mello, R.F. (2019) "On the Shattering Coefficient of Supervised Learning Algorithms" ; de Mello, R.F., Ponti, M.A. (2018, ISBN: 978-3319949888) "Machine Learning: A Practical Approach on the Statistical Learning Theory".
How to cite:
Rodrigo F. de Mello (2020). shattering: Estimate the Shattering Coefficient for a Particular Dataset. R package version 1.0.7, https://cran.r-project.org/web/packages/shattering. Accessed 27 Jan. 2025.
Previous versions and publish date:
Other packages that cited shattering R package
View shattering citation profile
Other R packages that shattering depends,
imports, suggests or enhances
Complete documentation for shattering
Functions, R codes and Examples using
the shattering R package
Some associated functions: apply_classifier . build_classifier . complexity_analysis . compress_space . equivalence_relation . estimate_number_hyperplanes . number_regions . shattering .
Some associated R codes: apply_classifier.R . build_classifier.R . complexity_analysis.R . compress_space.R . equivalence_relation.R . estimate_number_hyperplanes.R . number_regions.R . shattering.R . Full shattering package functions and examples
Downloads during the last 30 days
Get rewarded with contribution points by
helping add
Reviews / comments / questions /suggestions ↴↴↴
Today's Hot Picks in Authors and Packages
mgm
Estimation of k-Order time-varying Mixed Graphical Models and mixed VAR(p) models via elastic-net re ...
Download / Learn more Package Citations See dependency
Download / Learn more Package Citations See dependency
Maintainer: Jonas Haslbeck (view profile)
intccr
Semiparametric regression models on the cumulative incidence function for interval-censored competin ...
Download / Learn more Package Citations See dependency
Download / Learn more Package Citations See dependency
Maintainer: Jun Park (view profile)
quickcode
The NOT functions, 'R' tricks and a compilation of some simple quick plus often used 'R' codes to im ...
Download / Learn more Package Citations See dependency
Download / Learn more Package Citations See dependency
Maintainer: Obinna Obianom (view profile)
shinybrms
A graphical user interface (GUI) for fitting Bayesian regression
models using the package 'brms' wh ...
Download / Learn more Package Citations See dependency
Download / Learn more Package Citations See dependency
Maintainer: Frank Weber (view profile)
AssetCorr
Functions for the estimation of intra- and inter-cohort correlations in the Vasicek credit portfolio ...
Download / Learn more Package Citations See dependency
Download / Learn more Package Citations See dependency
Maintainer: Maximilian Nagl (view profile)
23,580
R Packages
204,057
Dependencies
63,980
Author Associations
23,561
Publication Badges
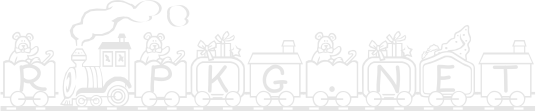