R package citation, R package reverse dependencies, R package scholars, install an r package from GitHub hy is package acceptance pending why is package undeliverable amazon why is package on hold dhl tour packages why in r package r and r package full form why is r free why r is bad which r package to install which r package has which r package which r package version which r package readxl which r package ggplot which r package fread which r package license where is package.json where is package-lock.json where is package.swift where is package explorer in eclipse where is package where is package manager unity where is package installer android where is package manager console in visual studio who r package which r package to install which r package version who is package who is package deal who is package design r and r package full form r and r package meaning what r package has what package r what is package in java what is package what is package-lock.json what is package in python what is package.json what is package installer do r package can't install r packages r can't find package r can't load package can't load xlsx package r can't install psych package r can't install sf package r Write if else in NONMEM pk pd
rsparse
View on CRAN: Click
here
Download and install rsparse package within the R console
Install from CRAN:
install.packages("rsparse")
Install from Github:
library("remotes")
install_github("cran/rsparse")
Install by package version:
library("remotes")
install_version("rsparse", "0.5.1")
Attach the package and use:
library("rsparse")
Maintained by
Dmitriy Selivanov
[Scholar Profile | Author Map]
[Scholar Profile | Author Map]
All associated links for this package
First Published: 2019-04-14
Latest Update: 2022-09-11
Description:
Implements many algorithms for statistical learning on sparse matrices - matrix factorizations, matrix completion, elastic net regressions, factorization machines. Also 'rsparse' enhances 'Matrix' package by providing methods for multithreaded <sparse, dense> matrix products and native slicing of the sparse matrices in Compressed Sparse Row (CSR) format. List of the algorithms for regression problems: 1) Elastic Net regression via Follow The Proximally-Regularized Leader (FTRL) Stochastic Gradient Descent (SGD), as per McMahan et al(, <doi:10.1145/2487575.2488200>) 2) Factorization Machines via SGD, as per Rendle (2010, <doi:10.1109/ICDM.2010.127>) List of algorithms for matrix factorization and matrix completion: 1) Weighted Regularized Matrix Factorization (WRMF) via Alternating Least Squares (ALS) - paper by Hu, Koren, Volinsky (2008, <doi:10.1109/ICDM.2008.22>) 2) Maximum-Margin Matrix Factorization via ALS, paper by Rennie, Srebro (2005, <doi:10.1145/1102351.1102441>) 3) Fast Truncated Singular Value Decomposition (SVD), Soft-Thresholded SVD, Soft-Impute matrix completion via ALS - paper by Hastie, Mazumder et al. (2014, <doi:10.48550/arXiv.1410.2596>) 4) Linear-Flow matrix factorization, from 'Practical linear models for large-scale one-class collaborative filtering' by Sedhain, Bui, Kawale et al (2016, ISBN:978-1-57735-770-4) 5) GlobalVectors (GloVe) matrix factorization via SGD, paper by Pennington, Socher, Manning (2014, <https://aclanthology.org/D14-1162/>) Package is reasonably fast and memory efficient - it allows to work with large datasets - millions of rows and millions of columns. This is particularly useful for practitioners working on recommender systems.
How to cite:
Dmitriy Selivanov (2019). rsparse: Statistical Learning on Sparse Matrices. R package version 0.5.1, https://cran.r-project.org/web/packages/rsparse
Previous versions and publish date:
Other packages that cited rsparse R package
View rsparse citation profile
Other R packages that rsparse depends,
imports, suggests or enhances
Functions, R codes and Examples using
the rsparse R package
Some associated functions: FTRL . FactorizationMachine . GloVe . LinearFlow . MatrixFactorizationRecommender . PureSVD . ScaleNormalize . WRMF . detect_number_omp_threads . metrics . movielens100k . soft_impute . train_test_split .
Some associated R codes: MatrixFactorizationRecommender.R . RcppExports.R . SoftALS.R . data.R . kmeans.R . metrics.R . model_FTRL.R . model_FactorizationMachine.R . model_GloVe.R . model_LinearFlow.R . model_PureSVD.R . model_RankMF.R . model_ScaleNormalize.R . model_WRMF.R . splr.R . utils.R . utils_SoftALS.R . zzz.R . Full rsparse package functions and examples
Downloads during the last 30 days
Get rewarded with contribution points by
helping add
Reviews / comments / questions /suggestions ↴↴↴
Today's Hot Picks in Authors and Packages
Deriv
R-based solution for symbolic differentiation. It admits user-defined function as well as function s ...
Download / Learn more Package Citations See dependency
Download / Learn more Package Citations See dependency
Maintainer: Serguei Sokol (view profile)
lgarch
Simulation and estimation of univariate and multivariate log-GARCH models. The main functions of the ...
Download / Learn more Package Citations See dependency
Download / Learn more Package Citations See dependency
Maintainer: Genaro Sucarrat (view profile)
bulletr
Analyze bullet lands using nonparametric methods. We provide a reading routine for x3p files (see &l ...
Download / Learn more Package Citations See dependency
Download / Learn more Package Citations See dependency
Maintainer: Eric Hare (view profile)
nextGenShinyApps
Nove responsive tools for designing and developing 'Shiny' dashboards and applications. The scripts ...
Download / Learn more Package Citations See dependency
Download / Learn more Package Citations See dependency
Maintainer: Obinna Obianom (view profile)
buildr
Working with reproducible reports or any other similar projects often require to run the script that ...
Download / Learn more Package Citations See dependency
Download / Learn more Package Citations See dependency
Maintainer: Jan Netik (view profile)
22,086
R Packages
187,731
Dependencies
55,244
Author Associations
22,087
Publication Badges
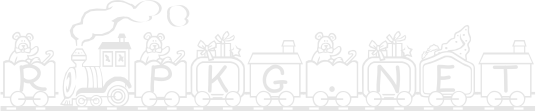
© Copyright 2022 - present. All right reserved, rpkg.net. Contact Us / Suggestions / Concerns