R package citation, R package reverse dependencies, R package scholars, install an r package from GitHub hy is package acceptance pending why is package undeliverable amazon why is package on hold dhl tour packages why in r package r and r package full form why is r free why r is bad which r package to install which r package has which r package which r package version which r package readxl which r package ggplot which r package fread which r package license where is package.json where is package-lock.json where is package.swift where is package explorer in eclipse where is package where is package manager unity where is package installer android where is package manager console in visual studio who r package which r package to install which r package version who is package who is package deal who is package design r and r package full form r and r package meaning what r package has what package r what is package in java what is package what is package-lock.json what is package in python what is package.json what is package installer do r package can't install r packages r can't find package r can't load package can't load xlsx package r can't install psych package r can't install sf package r Write if else in NONMEM pk pd
mlim
View on CRAN: Click
here
Download and install mlim package within the R console
Install from CRAN:
install.packages("mlim")
Install from Github:
library("remotes")
install_github("cran/mlim")
Install by package version:
library("remotes")
install_version("mlim", "0.3.0")
Attach the package and use:
library("mlim")
Maintained by
E. F. Haghish
[Scholar Profile | Author Map]
[Scholar Profile | Author Map]
All associated links for this package
First Published: 2022-08-13
Latest Update: 2022-12-16
Description:
Machine learning algorithms have been used for performing
single missing data imputation and most recently, multiple imputations.
However, this is the first attempt for using automated machine learning algorithms
for performing both
single and multiple imputation. Automated machine learning is a procedure for
fine-tuning the model automatic, performing a random search for a model that
results in less error, without overfitting the data. The main idea is
to allow the model to set its own parameters for imputing each variable separately
instead of setting fixed predefined parameters to impute all variables
of the dataset.
Using automated machine learning, the package fine-tunes an Elastic
Net (default) or Gradient Boosting, Random Forest, Deep Learning, Extreme Gradient Boosting,
or Stacked Ensemble machine learning model (from one or a combination of other
supported algorithms) for imputing the missing
observations. This procedure has been implemented for the
first time by this package and is expected to outperform other packages for
imputing missing data that do not fine-tune their models. The multiple imputation
is implemented via bootstrapping without letting the duplicated observations to
harm the cross-validation procedure, which is the way imputed variables are evaluated.
Most notably, the package implements automated procedure for handling imputing imbalanced
data (class rarity problem), which happens when a factor variable has a level that is far more
prevalent than the other(s). This is known to result in biased predictions, hence, biased
imputation of missing data. However, the autobalancing procedure ensures that instead of
focusing on maximizing accuracy (classification error) in imputing factor variables,
a fairer procedure and imputation method is practiced.
How to cite:
E. F. Haghish (2022). mlim: Single and Multiple Imputation with Automated Machine Learning. R package version 0.3.0, https://cran.r-project.org/web/packages/mlim. Accessed 03 Dec. 2024.
Previous versions and publish date:
Other packages that cited mlim R package
View mlim citation profile
Other R packages that mlim depends,
imports, suggests or enhances
Complete documentation for mlim
Functions, R codes and Examples using
the mlim R package
Some associated functions: charity . manifest . mlim.error . mlim.mids . mlim.na . mlim . mlim.preimpute . mlim.summarize .
Some associated R codes: addNA.R . algoSelector.R . bootstrapCV.R . bootstrapWeight.R . charity.R . checkNconvert.R . defaultCV.R . extractMetrics.R . factmem.R . getDigits.R . getMetrics.R . h2o.check.R . init.R . is.imbalanced.R . is.valid.R . iterate.R . iterationNextVar.R . iteration_loop.R . javaServer.R . manifest.R . matching.R . meanmode.R . miss_per_class_error.R . missclass.R . missrank.R . mlim.R . mlim.error.R . mlim.mids.R . mlim.na.R . mlim.postimpute.R . mlim.preimpute.R . mlim.shuffle.R . mlim.summarize.R . normalize.R . nrmse.R . revert.R . selectVariables.R . server.check.R . stochasticFactorImpute.R . stoppingCriteria.R . syntaxProcessing.R . threeDots.R . zzz.R . Full mlim package functions and examples
Downloads during the last 30 days
Get rewarded with contribution points by
helping add
Reviews / comments / questions /suggestions ↴↴↴
Today's Hot Picks in Authors and Packages
CRANsearcher
One of the strengths of R is its vast package ecosystem. Indeed, R packages extend from visualizatio ...
Download / Learn more Package Citations See dependency
Download / Learn more Package Citations See dependency
Maintainer: Agustin Calatroni (view profile)
donut
Finds the k nearest neighbours in a dataset of specified points,
adding the option to wrap certain ...
Download / Learn more Package Citations See dependency
Download / Learn more Package Citations See dependency
Maintainer: Paul J. Northrop (view profile)
FSInteract
Performs fast detection of interactions in large-scale data using the
method of random intersection ...
Download / Learn more Package Citations See dependency
Download / Learn more Package Citations See dependency
Maintainer: Rajen D. Shah (view profile)
EDOtrans
A data transformation method which takes into account the special property of scale non-invariance w ...
Download / Learn more Package Citations See dependency
Download / Learn more Package Citations See dependency
Maintainer: Jorn Lotsch (view profile)
telemac
An R interface to the TELEMAC suite for modelling
of free surface flow. This includes methods for m ...
Download / Learn more Package Citations See dependency
Download / Learn more Package Citations See dependency
Maintainer: Tobias Pilz (view profile)
r2resize
Automatic resizing toolbar for containers, images and tables. Various resizable or expandable contai ...
Download / Learn more Package Citations See dependency
Download / Learn more Package Citations See dependency
Maintainer: Obinna Obianom (view profile)
23,310
R Packages
200,798
Dependencies
63,203
Author Associations
23,278
Publication Badges
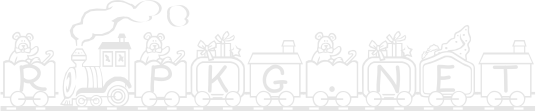