R package citation, R package reverse dependencies, R package scholars, install an r package from GitHub hy is package acceptance pending why is package undeliverable amazon why is package on hold dhl tour packages why in r package r and r package full form why is r free why r is bad which r package to install which r package has which r package which r package version which r package readxl which r package ggplot which r package fread which r package license where is package.json where is package-lock.json where is package.swift where is package explorer in eclipse where is package where is package manager unity where is package installer android where is package manager console in visual studio who r package which r package to install which r package version who is package who is package deal who is package design r and r package full form r and r package meaning what r package has what package r what is package in java what is package what is package-lock.json what is package in python what is package.json what is package installer do r package can't install r packages r can't find package r can't load package can't load xlsx package r can't install psych package r can't install sf package r Write if else in NONMEM pk pd
interpret
View on CRAN: Click
here
Download and install interpret package within the R console
Install from CRAN:
install.packages("interpret")
Install from Github:
library("remotes")
install_github("cran/interpret")
Install by package version:
library("remotes")
install_version("interpret", "0.1.33")
Attach the package and use:
library("interpret")
Maintained by
Rich Caruana
[Scholar Profile | Author Map]
[Scholar Profile | Author Map]
All associated links for this package
First Published: 2019-10-06
Latest Update: 2023-01-27
Description:
Package for training interpretable machine learning models. Historically, the most interpretable machine learning models were not very accurate, and the most accurate models were not very interpretable. Microsoft Research has developed an algorithm called the Explainable Boosting Machine (EBM) which has both high accuracy and interpretable characteristics. EBM uses machine learning techniques like bagging and boosting to breathe new life into traditional GAMs (Generalized Additive Models). This makes them as accurate as random forests and gradient boosted trees, and also enhances their intelligibility and editability. Details on the EBM algorithm can be found in the paper by Rich Caruana, Yin Lou, Johannes Gehrke, Paul Koch, Marc Sturm, and Noemie Elhadad (2015, ).
How to cite:
Rich Caruana (2019). interpret: Fit Interpretable Machine Learning Models. R package version 0.1.33, https://cran.r-project.org/web/packages/interpret
Previous versions and publish date:
Other packages that cited interpret R package
View interpret citation profile
Other R packages that interpret depends,
imports, suggests or enhances
Functions, R codes and Examples using
the interpret R package
Some associated functions: ebm_classify . ebm_predict_proba . ebm_show .
Some associated R codes: binning.R . booster.R . dataset.R . ebm.R . interaction_detector.R . random_numbers.R . sampling.R . Full interpret package functions and examples
Downloads during the last 30 days
Get rewarded with contribution points by
helping add
Reviews / comments / questions /suggestions ↴↴↴
Today's Hot Picks in Authors and Packages
QuantileNPCI
Based on Alan D. Hutson (1999) , "Calculating nonparametric confidence i ...
Download / Learn more Package Citations See dependency
Download / Learn more Package Citations See dependency
Maintainer: Li Yan (view profile)
FMCCSD
Current status data abounds
in the field of epidemiology and public health, where the only observab ...
Download / Learn more Package Citations See dependency
Download / Learn more Package Citations See dependency
Maintainer: Tong Wang (view profile)
cdcfluview
The 'U.S.' Centers for Disease Control and Prevention (CDC) maintain
a portal ...
Download / Learn more Package Citations See dependency
Download / Learn more Package Citations See dependency
Maintainer: Bob Rudis (view profile)
srm
Provides functionality for structural equation modeling for the social relations model (Kenny & ...
Download / Learn more Package Citations See dependency
Download / Learn more Package Citations See dependency
Maintainer: Alexander Robitzsch (view profile)
bizicount
Maximum likelihood estimation of copula-based zero-inflated
(and non-inflated) Poisson and negative ...
Download / Learn more Package Citations See dependency
Download / Learn more Package Citations See dependency
Maintainer: John Niehaus (view profile)
r2resize
Automatic resizing toolbar for containers, images and tables. Various resizable or expandable contai ...
Download / Learn more Package Citations See dependency
Download / Learn more Package Citations See dependency
Maintainer: Obinna Obianom (view profile)
22,114
R Packages
188,080
Dependencies
55,244
Author Associations
22,115
Publication Badges
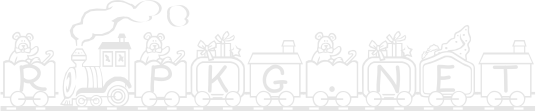
© Copyright 2022 - present. All right reserved, rpkg.net. Contact Us / Suggestions / Concerns