R package citation, R package reverse dependencies, R package scholars, install an r package from GitHub hy is package acceptance pending why is package undeliverable amazon why is package on hold dhl tour packages why in r package r and r package full form why is r free why r is bad which r package to install which r package has which r package which r package version which r package readxl which r package ggplot which r package fread which r package license where is package.json where is package-lock.json where is package.swift where is package explorer in eclipse where is package where is package manager unity where is package installer android where is package manager console in visual studio who r package which r package to install which r package version who is package who is package deal who is package design r and r package full form r and r package meaning what r package has what package r what is package in java what is package what is package-lock.json what is package in python what is package.json what is package installer do r package can't install r packages r can't find package r can't load package can't load xlsx package r can't install psych package r can't install sf package r Write if else in NONMEM pk pd
gausscov
View on CRAN: Click
here
Download and install gausscov package within the R console
Install from CRAN:
install.packages("gausscov")
Install from Github:
library("remotes")
install_github("cran/gausscov")
Install by package version:
library("remotes")
install_version("gausscov", "1.1.3")
Attach the package and use:
library("gausscov")
Maintained by
Laurie Davies
[Scholar Profile | Author Map]
[Scholar Profile | Author Map]
All associated links for this package
First Published: 2019-06-18
Latest Update: 2024-03-04
Description:
The standard linear regression theory whether frequentist or Bayesian is based on an 'assumed (revealed?) truth' (John Tukey) attitude to models. This is reflected in the language of statistical inference which involves a concept of truth, for example confidence intervals, hypothesis testing and consistency. The motivation behind this package was to remove the word true from the theory and practice of linear regression and to replace it by approximation. The approximations considered are the least squares approximations. An approximation is called valid if it contains no irrelevant covariates. This is operationalized using the concept of a Gaussian P-value which is the probability that pure Gaussian noise is better in term of least squares than the covariate. The precise definition given in the paper, it is intuitive and requires only four simple equations. Its overwhelming advantage compared with a standard F P-value is that is is exact and valid whatever the data. In contrast F P-values are only valid for specially designed simulations. Given this a valid approximation is one where all the Gaussian P-values are less than a threshold p0 specified by the statistician, in this package with the default value 0.01. This approximations approach is not only much simpler it is overwhelmingly better than the standard model based approach. The will be demonstrated using six real data sets, four from high dimensional regression and two from vector autoregression. The simplicity and superiority of Gaussian P-values derive from their universal exactness and validity. This is in complete contrast to standard F P-values which are valid only for carefully designed simulations. The function f1st is the most important function. It is a greedy forward selection procedure which results in either just one or no approximations which may however not be valid. If the size is less than than a threshold with default value 21 then an all subset procedure is called which returns the best valid subset. A good default start is f1st(y,x,kmn=15) The best function for returning multiple approximations is f3st which repeatedly calls f1st. For more information see the web site below and the accompanying papers: L. Davies and L. Duembgen, "Covariate Selection Based on a Model-free Approach to Linear Regression with Exact Probabilities", 2022, . L. Davies, "An Approximation Based Theory of Linear Regression", 2024, .
How to cite:
Laurie Davies (2019). gausscov: The Gaussian Covariate Method for Variable Selection. R package version 1.1.3, https://cran.r-project.org/web/packages/gausscov. Accessed 21 Nov. 2024.
Previous versions and publish date:
0.0.1 (2019-06-18 17:30), 0.0.2 (2019-09-11 22:50), 0.0.3 (2020-02-13 19:40), 0.0.4 (2020-08-01 18:20), 0.0.5 (2020-08-28 09:30), 0.0.6 (2020-09-05 11:30), 0.0.7 (2020-09-14 14:00), 0.0.8 (2020-10-21 17:00), 0.0.9 (2020-11-07 14:20), 0.0.10 (2020-11-22 13:40), 0.0.11 (2021-01-13 11:50), 0.0.12 (2021-01-27 17:00), 0.0.13 (2021-02-26 14:30), 0.1.0 (2021-03-28 19:10), 0.1.1 (2021-04-30 10:00), 0.1.2 (2021-12-16 15:30), 0.1.3 (2021-12-19 03:10), 0.1.4 (2022-01-17 09:42), 0.1.5 (2022-02-11 14:40), 0.1.6 (2022-03-14 15:50), 0.1.7 (2022-04-26 17:50), 0.1.8 (2022-06-26 18:50), 0.1.9 (2022-11-12 17:40), 1.0.0 (2022-12-08 11:10), 1.0.1 (2023-01-17 10:40), 1.0.2 (2023-02-02 19:10), 1.0.3 (2023-10-11 21:40), 1.1.0 (2024-02-29 13:22), 1.1.1 (2024-03-04 20:20), 1.1.2 (2024-03-19 17:20)
Other packages that cited gausscov R package
View gausscov citation profile
Other R packages that gausscov depends,
imports, suggests or enhances
Complete documentation for gausscov
Functions, R codes and Examples using
the gausscov R package
Some associated functions: abcql . boston . decode . f1st . f2st . f3st . f3sti . fasb . fdecode . fgeninter . fgentrig . fgr1st . fgr2st . flag . fpval . fselect . fundr . fvauto . leukemia . mel_temp . redwine . simgpval . snspt .
Some associated R codes: decode.R . f1st.R . f2st.R . f3st.R . f3sti.R . fasb.R . fdecode.R . fgeninter.R . fgentrig.R . fgr1st.R . fgr2st.R . flag.R . fpval.R . fselect.R . fundr.R . fvauto.R . simgpval.R . Full gausscov package functions and examples
Downloads during the last 30 days
Get rewarded with contribution points by
helping add
Reviews / comments / questions /suggestions ↴↴↴
Today's Hot Picks in Authors and Packages
SCBiclust
Identifies a bicluster, a submatrix of the data such that the features and observations within the s ...
Download / Learn more Package Citations See dependency
Download / Learn more Package Citations See dependency
Maintainer: Erika S. Helgeson (view profile)
r2resize
Automatic resizing toolbar for containers, images and tables. Various resizable or expandable contai ...
Download / Learn more Package Citations See dependency
Download / Learn more Package Citations See dependency
Maintainer: Obinna Obianom (view profile)
deductive
Attempt to repair inconsistencies and missing values in
data records by using information from vali ...
Download / Learn more Package Citations See dependency
Download / Learn more Package Citations See dependency
Maintainer: Mark van der Loo (view profile)
RcppHNSW
'Hnswlib' is a C++ library for Approximate Nearest Neighbors.
This package provides a minimal R int ...
Download / Learn more Package Citations See dependency
Download / Learn more Package Citations See dependency
Maintainer: James Melville (view profile)
pkgdepends
Find recursive dependencies of 'R' packages from various
sources. Solve the dependencies to obtain ...
Download / Learn more Package Citations See dependency
Download / Learn more Package Citations See dependency
Maintainer: Gábor Csárdi (view profile)
kgschart
Restore underlining numeric data from rating history graph of
KGS (an online platform of the game o ...
Download / Learn more Package Citations See dependency
Download / Learn more Package Citations See dependency
Maintainer: Kota Mori (view profile)
23,229
R Packages
199,929
Dependencies
62,984
Author Associations
23,230
Publication Badges
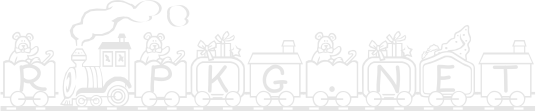