R package citation, R package reverse dependencies, R package scholars, install an r package from GitHub hy is package acceptance pending why is package undeliverable amazon why is package on hold dhl tour packages why in r package r and r package full form why is r free why r is bad which r package to install which r package has which r package which r package version which r package readxl which r package ggplot which r package fread which r package license where is package.json where is package-lock.json where is package.swift where is package explorer in eclipse where is package where is package manager unity where is package installer android where is package manager console in visual studio who r package which r package to install which r package version who is package who is package deal who is package design r and r package full form r and r package meaning what r package has what package r what is package in java what is package what is package-lock.json what is package in python what is package.json what is package installer do r package can't install r packages r can't find package r can't load package can't load xlsx package r can't install psych package r can't install sf package r Write if else in NONMEM pk pd
autohrf
View on CRAN: Click
here
Download and install autohrf package within the R console
Install from CRAN:
install.packages("autohrf")
Install from Github:
library("remotes")
install_github("cran/autohrf")
Install by package version:
library("remotes")
install_version("autohrf", "1.1.3")
Attach the package and use:
library("autohrf")
Maintained by
Jure Demšar
[Scholar Profile | Author Map]
[Scholar Profile | Author Map]
All associated links for this package
First Published: 2022-07-21
Latest Update: 2023-02-15
Description:
Analysis of task-related functional magnetic resonance imaging (fMRI) activity at the level of individual participants is commonly based on general linear modelling (GLM) that allows us to estimate to what extent the blood oxygenation level dependent (BOLD) signal can be explained by task response predictors specified in the GLM model. The predictors are constructed by convolving the hypothesised timecourse of neural activity with an assumed hemodynamic response function (HRF). To get valid and precise estimates of task response, it is important to construct a model of neural activity that best matches actual neuronal activity. The construction of models is most often driven by predefined assumptions on the components of brain activity and their duration based on the task design and specific aims of the study. However, our assumptions about the onset and duration of component processes might be wrong and can also differ across brain regions. This can result in inappropriate or suboptimal models, bad fitting of the model to the actual data and invalid estimations of brain activity. Here we present an approach in which theoretically driven models of task response are used to define constraints based on which the final model is derived computationally using the actual data. Specifically, we developed 'autohrf'
How to cite:
Jure Demšar (2022). autohrf: Automated Generation of Data-Informed GLM Models in Task-Based fMRI Data Analysis. R package version 1.1.3, https://cran.r-project.org/web/packages/autohrf
Previous versions and publish date:
Other packages that cited autohrf R package
View autohrf citation profile
Other R packages that autohrf depends,
imports, suggests or enhances
Functions, R codes and Examples using
the autohrf R package
Some associated functions: autohrf-datasets . autohrf . convolve_events . convolve_hrf . create_boynton_hrf . create_child . create_first_generation . create_new_generation . create_spm_hrf . downsample . evaluate_model . fit_to_constraints . get_best_models . get_parents . plot_best_models . plot_events . plot_fitness . plot_model . run_model .
Some associated R codes: data.R . events.R . hrf.R . modelling.R . signal.R . Full autohrf package functions and examples
Downloads during the last 30 days
Get rewarded with contribution points by
helping add
Reviews / comments / questions /suggestions ↴↴↴
Today's Hot Picks in Authors and Packages
gmt
Interface between the GMT map-making software and R, enabling the
user to manipulate geographic dat ...
Download / Learn more Package Citations See dependency
Download / Learn more Package Citations See dependency
Maintainer: Arni Magnusson (view profile)
EasyDescribe
Descriptive Statistics is essential for publishing articles. This package can perform
descriptive s ...
Download / Learn more Package Citations See dependency
Download / Learn more Package Citations See dependency
Maintainer: Xiuquan Nie (view profile)
hydropeak
An important environmental impact on running water ecosystems
is caused by hydropeaking - the disco ...
Download / Learn more Package Citations See dependency
Download / Learn more Package Citations See dependency
Maintainer: Bettina Grün (view profile)
nextGenShinyApps
Nove responsive tools for designing and developing 'Shiny' dashboards and applications. The scripts ...
Download / Learn more Package Citations See dependency
Download / Learn more Package Citations See dependency
Maintainer: Obinna Obianom (view profile)
rankdist
Implements distance based probability models for ranking data.
The supported distance metrics inclu ...
Download / Learn more Package Citations See dependency
Download / Learn more Package Citations See dependency
Maintainer: Zhaozhi Qian (view profile)
trapezoid
The trapezoid package provides 'dtrapezoid', 'ptrapezoid', 'qtrapezoid', and 'rtrapezoid' functions ...
Download / Learn more Package Citations See dependency
Download / Learn more Package Citations See dependency
Maintainer: Jeremy Thoms Hetzel (view profile)
22,187
R Packages
188,753
Dependencies
55,244
Author Associations
22,188
Publication Badges
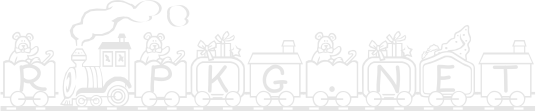
© Copyright 2022 - present. All right reserved, rpkg.net. Contact Us / Suggestions / Concerns