R package citation, R package reverse dependencies, R package scholars, install an r package from GitHub hy is package acceptance pending why is package undeliverable amazon why is package on hold dhl tour packages why in r package r and r package full form why is r free why r is bad which r package to install which r package has which r package which r package version which r package readxl which r package ggplot which r package fread which r package license where is package.json where is package-lock.json where is package.swift where is package explorer in eclipse where is package where is package manager unity where is package installer android where is package manager console in visual studio who r package which r package to install which r package version who is package who is package deal who is package design r and r package full form r and r package meaning what r package has what package r what is package in java what is package what is package-lock.json what is package in python what is package.json what is package installer do r package can't install r packages r can't find package r can't load package can't load xlsx package r can't install psych package r can't install sf package r Write if else in NONMEM pk pd
SimCorrMix
View on CRAN: Click
here
Download and install SimCorrMix package within the R console
Install from CRAN:
install.packages("SimCorrMix")
Install from Github:
library("remotes")
install_github("cran/SimCorrMix")
Install by package version:
library("remotes")
install_version("SimCorrMix", "0.1.1")
Attach the package and use:
library("SimCorrMix")
Maintained by
Allison Cynthia Fialkowski
[Scholar Profile | Author Map]
[Scholar Profile | Author Map]
All associated links for this package
First Published: 2018-02-26
Latest Update: 2018-07-01
Description:
Generate continuous (normal, non-normal, or mixture distributions), binary, ordinal, and count (regular or zero-inflated, Poisson or Negative Binomial) variables with a specified correlation matrix, or one continuous variable with a mixture distribution.This package can be used to simulate data sets that mimic real-world clinical or genetic data sets (i.e., plasmodes, as in Vaughan et al., 2009 <doi:10.1016/j.csda.2008.02.032>).The methods extend those found in the 'SimMultiCorrData' R package.Standard normal variables with an imposed intermediate correlation matrix are transformed to generate the desired distributions. Continuous variables are simulated using either Fleishman (1978)'s third order <doi:10.1007/BF02293811> or Headrick (2002)'s fifth order <doi:10.1016/S0167-9473(02)00072-5> polynomial transformation method (the power method transformation, PMT).Non-mixture distributions require the user to specify mean, variance, skewness, standardized kurtosis, and standardized fifth and sixth cumulants.Mixture distributions require these inputs for the component distributions plus the mixing probabilities.Simulation occurs at the component level for continuous mixture distributions.The target correlation matrix is specified in terms of correlations with components of continuous mixture variables.These components are transformed into the desired mixture variables using random multinomial variables based on the mixing probabilities.However, the package provides functions to approximate expected correlations with continuous mixture variables given target correlations with the components. Binary and ordinal variables are simulated using a modification of ordsample() in package 'GenOrd'. Count variables are simulated using the inverse CDF method.There are two simulation pathways which calculate intermediate correlations involving count variables differently. Correlation Method 1 adapts Yahav and Shmueli's 2012 method <doi:10.1002/asmb.901> and performs best with large count variable means and positive correlations or small means and negative correlations.Correlation Method 2 adapts Barbiero and Ferrari's 2015 modification of the 'GenOrd' package <doi:10.1002/asmb.2072> and performs best under the opposite scenarios.The optional error loop may be used to improve the accuracy of the final correlation matrix.The package also contains functions to calculate the standardized cumulants of continuous mixture distributions, check parameter inputs, calculate feasible correlation boundaries, and summarize and plot simulated variables.
How to cite:
Allison Cynthia Fialkowski (2018). SimCorrMix: Simulation of Correlated Data with Multiple Variable Types Including Continuous and Count Mixture Distributions. R package version 0.1.1, https://cran.r-project.org/web/packages/SimCorrMix. Accessed 22 Dec. 2024.
Previous versions and publish date:
0.1.0 (2018-02-26 20:04)
Other packages that cited SimCorrMix R package
View SimCorrMix citation profile
Other R packages that SimCorrMix depends,
imports, suggests or enhances
Complete documentation for SimCorrMix
Functions, R codes and Examples using
the SimCorrMix R package
Some associated functions: SimCorrMix . calc_mixmoments . contmixvar1 . corr_error . corrvar . corrvar2 . intercorr . intercorr2 . intercorr_cat_nb . intercorr_cat_pois . intercorr_cont . intercorr_cont_nb . intercorr_cont_nb2 . intercorr_cont_pois . intercorr_cont_pois2 . intercorr_nb . intercorr_pois . intercorr_pois_nb . maxcount_support . norm_ord . ord_norm . plot_simpdf_theory . plot_simtheory . rho_M1M2 . rho_M1Y . summary_var . validcorr . validcorr2 . validpar .
Some associated R codes: SimCorrMix.R . calc_mixmoments.R . contmixvar1.R . corr_error.R . corrvar.R . corrvar2.R . intercorr.R . intercorr2.R . intercorr_cat_nb.R . intercorr_cat_pois.R . intercorr_cont.R . intercorr_cont_nb.R . intercorr_cont_nb2.R . intercorr_cont_pois.R . intercorr_cont_pois2.R . intercorr_nb.R . intercorr_pois.R . intercorr_pois_nb.R . maxcount_support.R . norm_ord.R . ord_norm.R . plot_simpdf_theory.R . plot_simtheory.R . rho_M1M2.R . rho_M1Y.R . summary_var.R . validcorr.R . validcorr2.R . validpar.R . Full SimCorrMix package functions and examples
Downloads during the last 30 days
Get rewarded with contribution points by
helping add
Reviews / comments / questions /suggestions ↴↴↴
Today's Hot Picks in Authors and Packages
composits
A compositional multivariate and univariate time series outlier ensemble.It uses the four R packages ...
Download / Learn more Package Citations See dependency
Download / Learn more Package Citations See dependency
Maintainer: Sevvandi Kandanaarachchi (view profile)
Rfast2
A collection of fast statistical and utility functions for data analysis. Functions for regression, ...
Download / Learn more Package Citations See dependency
Download / Learn more Package Citations See dependency
Maintainer: Manos Papadakis (view profile)
quickcode
The NOT functions, 'R' tricks and a compilation of some simple quick plus often used 'R' codes to im ...
Download / Learn more Package Citations See dependency
Download / Learn more Package Citations See dependency
Maintainer: Obinna Obianom (view profile)
wordspace
An interactive laboratory for research on distributional semantic models ('DSM', see < ...
Download / Learn more Package Citations See dependency
Download / Learn more Package Citations See dependency
Maintainer: Stephanie Evert (view profile)
LOGANTree
Enables researchers to model log-file data from computer-based assessments using machine-learning te ...
Download / Learn more Package Citations See dependency
Download / Learn more Package Citations See dependency
Maintainer: Qi Qin (view profile)
dmlalg
Implementation of double machine learning (DML) algorithms in R,
based on Emmenegger and Buehlmann ...
Download / Learn more Package Citations See dependency
Download / Learn more Package Citations See dependency
Maintainer: Corinne Emmenegger (view profile)
23,394
R Packages
201,798
Dependencies
63,416
Author Associations
23,395
Publication Badges
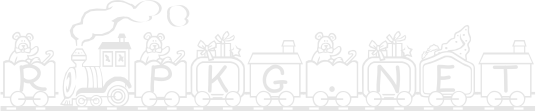