R package citation, R package reverse dependencies, R package scholars, install an r package from GitHub hy is package acceptance pending why is package undeliverable amazon why is package on hold dhl tour packages why in r package r and r package full form why is r free why r is bad which r package to install which r package has which r package which r package version which r package readxl which r package ggplot which r package fread which r package license where is package.json where is package-lock.json where is package.swift where is package explorer in eclipse where is package where is package manager unity where is package installer android where is package manager console in visual studio who r package which r package to install which r package version who is package who is package deal who is package design r and r package full form r and r package meaning what r package has what package r what is package in java what is package what is package-lock.json what is package in python what is package.json what is package installer do r package can't install r packages r can't find package r can't load package can't load xlsx package r can't install psych package r can't install sf package r Write if else in NONMEM pk pd
ICompELM
View on CRAN: Click
here
Download and install ICompELM package within the R console
Install from CRAN:
install.packages("ICompELM")
Install from Github:
library("remotes")
install_github("cran/ICompELM")
Install by package version:
library("remotes")
install_version("ICompELM", "0.1.0")
Attach the package and use:
library("ICompELM")
Maintained by
Saikath Das
[Scholar Profile | Author Map]
[Scholar Profile | Author Map]
All associated links for this package
10.32614/CRAN.package.ICompELM . ICompELM results . ICompELM.pdf . ICompELM_0.1.0.tar.gz . ICompELM_0.1.0.zip . ICompELM_0.1.0.zip . ICompELM_0.1.0.zip . ICompELM_0.1.0.tgz . ICompELM_0.1.0.tgz . ICompELM_0.1.0.tgz . ICompELM_0.1.0.tgz . ICompELM_0.1.0.tgz . ICompELM_0.1.0.tgz . https://CRAN.R-project.org/package=ICompELM .
First Published: 2024-06-10
Latest Update: 2024-06-10
Description:
Single Layer Feed-forward Neural networks (SLFNs) have many applications in various fields of statistical modelling, especially for time-series forecasting. However, there are some major disadvantages of training such networks via the widely accepted 'gradient-based backpropagation' algorithm, such as convergence to local minima, dependencies on learning rate and large training time. These concerns were addressed by Huang et al. (2006) <doi:10.1016/j.neucom.2005.12.126>, wherein they introduced the Extreme Learning Machine (ELM), an extremely fast learning algorithm for SLFNs which randomly chooses the weights connecting input and hidden nodes and analytically determines the output weights of SLFNs. It shows good generalized performance, but is still subject to a high degree of randomness. To mitigate this issue, this package uses a dimensionality reduction technique given in Hyvarinen (1999) <doi:10.1109/72.761722>, namely, the Independent Component Analysis (ICA) to determine the input-hidden connections and thus, remove any sort of randomness from the algorithm. This leads to a robust, fast and stable ELM model. Using functions within this package, the proposed model can also be compared with an existing alternative based on the Principal Component Analysis (PCA) algorithm given by Pearson (1901) <doi:10.1080/14786440109462720>, i.e., the PCA based ELM model given by Castano et al. (2013) <doi:10.1007/s11063-012-9253-x>, from which the implemented ICA based algorithm is greatly inspired.
How to cite:
Saikath Das (2024). ICompELM: Independent Component Analysis Based Extreme Learning Machine. R package version 0.1.0, https://cran.r-project.org/web/packages/ICompELM. Accessed 07 Apr. 2025.
Previous versions and publish date:
No previous versions
Other packages that cited ICompELM R package
View ICompELM citation profile
Other R packages that ICompELM depends,
imports, suggests or enhances
Complete documentation for ICompELM
Functions, R codes and Examples using
the ICompELM R package
Full ICompELM package
functions and examples
Downloads during the last 30 days
Today's Hot Picks in Authors and Packages
letsR
Handling, processing, and analyzing geographic
data on species' distributions and environmental var ...
Download / Learn more Package Citations See dependency
Download / Learn more Package Citations See dependency
Maintainer: Bruno Vilela (view profile)
r2resize
Automatic resizing toolbar for containers, images and tables. Various resizable or expandable contai ...
Download / Learn more Package Citations See dependency
Download / Learn more Package Citations See dependency
Maintainer: Obinna Obianom (view profile)
probout
Estimates unsupervised outlier probabilities for multivariate numeric data with many observations fr ...
Download / Learn more Package Citations See dependency
Download / Learn more Package Citations See dependency
Maintainer: Chris Fraley (view profile)
nutriNetwork
Statistical tool for learning the structure of direct associations among variables for
continuous d ...
Download / Learn more Package Citations See dependency
Download / Learn more Package Citations See dependency
Maintainer: Pariya Behrouzi (view profile)
MetaAnalyser
An interactive application to visualise meta-analysis data as a
physical weighing machine. The inte ...
Download / Learn more Package Citations See dependency
Download / Learn more Package Citations See dependency
Maintainer: Christopher Jackson (view profile)
ASMap
Functions for Accurate and Speedy linkage map construction, manipulation and diagnosis of Doubled Ha ...
Download / Learn more Package Citations See dependency
Download / Learn more Package Citations See dependency
Maintainer: Julian Taylor (view profile)
24,012
R Packages
207,311
Dependencies
64,867
Author Associations
24,013
Publication Badges
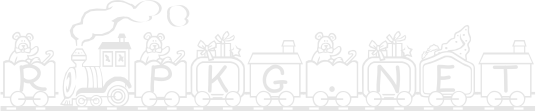